What is LlamaParse?
LlamaParse is a proprietary parsing service that is incredibly good at parsing PDFs with complex tables into a well-structured markdown format.
It directly integrates with LlamaIndex ingestion and retrieval to let you build retrieval over complex, semi-structured documents. It is promised to be able to answer complex questions that weren’t possible previously. This service is available in a public preview mode: available to everyone, but with a usage limit (1k pages per day) with 7,000 free pages per week. Then $0.003 per page ($3 per 1,000 pages). It operates as a standalone service that can also be plugged into the managed ingestion and retrieval API
Currently, LlamaParse primarily supports PDFs with tables, but they are also building out better support for figures, and an expanded set of the most popular document types: .docx, .pptx, .html as a part of the next enhancements.
Code Implementation:
- Install required dependencies:
a) Create requirements.txt in the root of your project and add these dependencies:
o llama-index
o llama-parse
o python-dotenv
b) Run the command from terminal “pip install -r requirements.txt” to download and install the above-mentioned dependencies from the folder where you have “requirements.txt”. - Set up the environment variables:
a) Create a “.env” file in the root of your project.
b) Add these variables there:
LLAMA_CLOUD_API_KEY = “PassYourLLAMACloudAPIKey”
OPENAI_API_KEY = “PassYourOpenAIAPIKey” - Create a folder named “data” in the root of your project and add a pdf file that you would like to read/parse like:
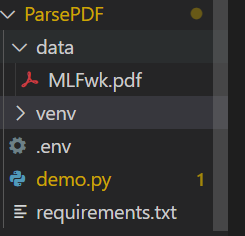
4. Create a file “demo.py” and add this code:
Note* I have added 2 queries at the end related to the pdf that I have provided under “data” folder, you should update queries based on the pdf that you are keeping under “data” folder.
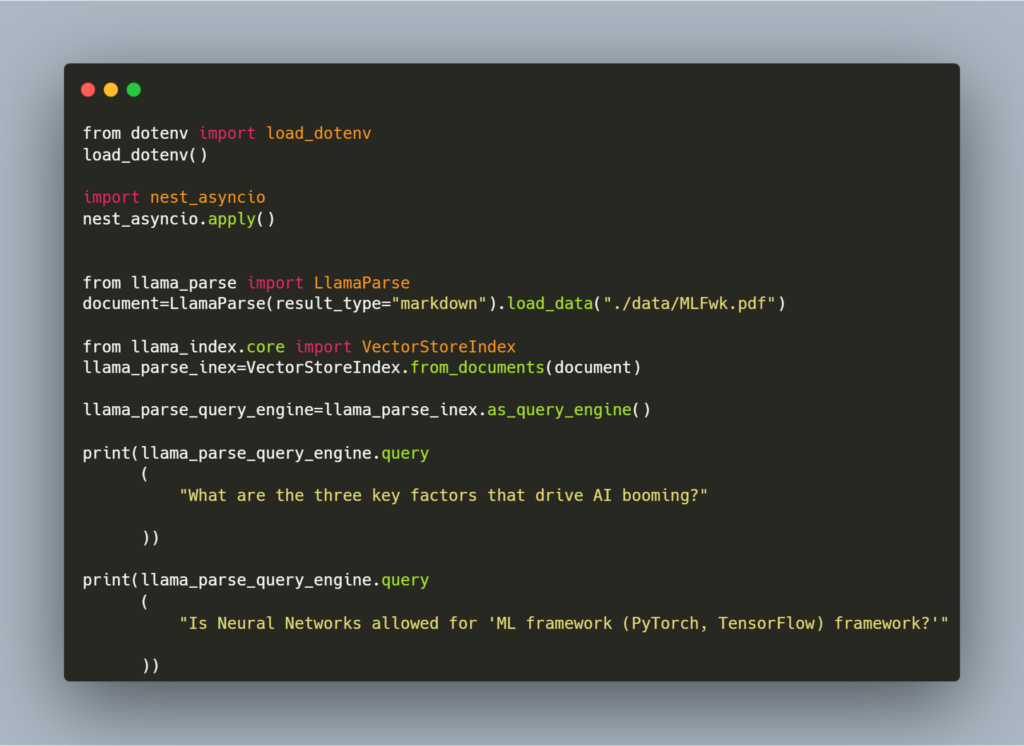
from dotenv import load_dotenv
load_dotenv()
import nest_asyncio
nest_asyncio.apply()
from llama_parse import LlamaParse
document=LlamaParse(result_type="markdown").load_data("./data/MLFwk.pdf")
from llama_index.core import VectorStoreIndex
llama_parse_inex=VectorStoreIndex.from_documents(document)
llama_parse_query_engine=llama_parse_inex.as_query_engine()
print(llama_parse_query_engine.query
(
"What are the three key factors that drive AI booming?"
))
print(llama_parse_query_engine.query
(
"Is Neural Networks allowed for 'ML framework (PyTorch, TensorFlow) framework?'"
))
- Execute the demo.py using the command “python .\demo.py”

Just for your reference, it gave the correct response as this was there in my “MLFwk.pdf” that I kept under the data folder:
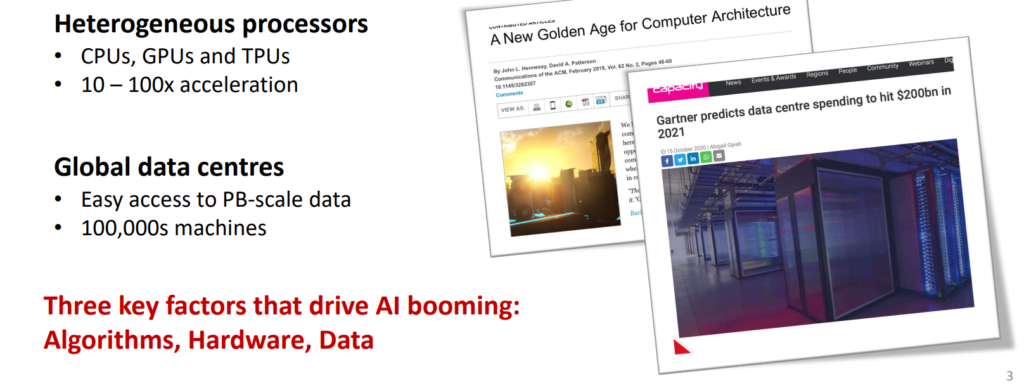

Conclusion:
From the above implementation, we can conclude that LlamaParse is incredibly good at parsing PDFs with complex tables into a well-structured markdown format.